Life Cycle Value Tradeoff of Noise Mitigating Measures Using BIM-Simulation and Multi-Criteria Decision Modeling
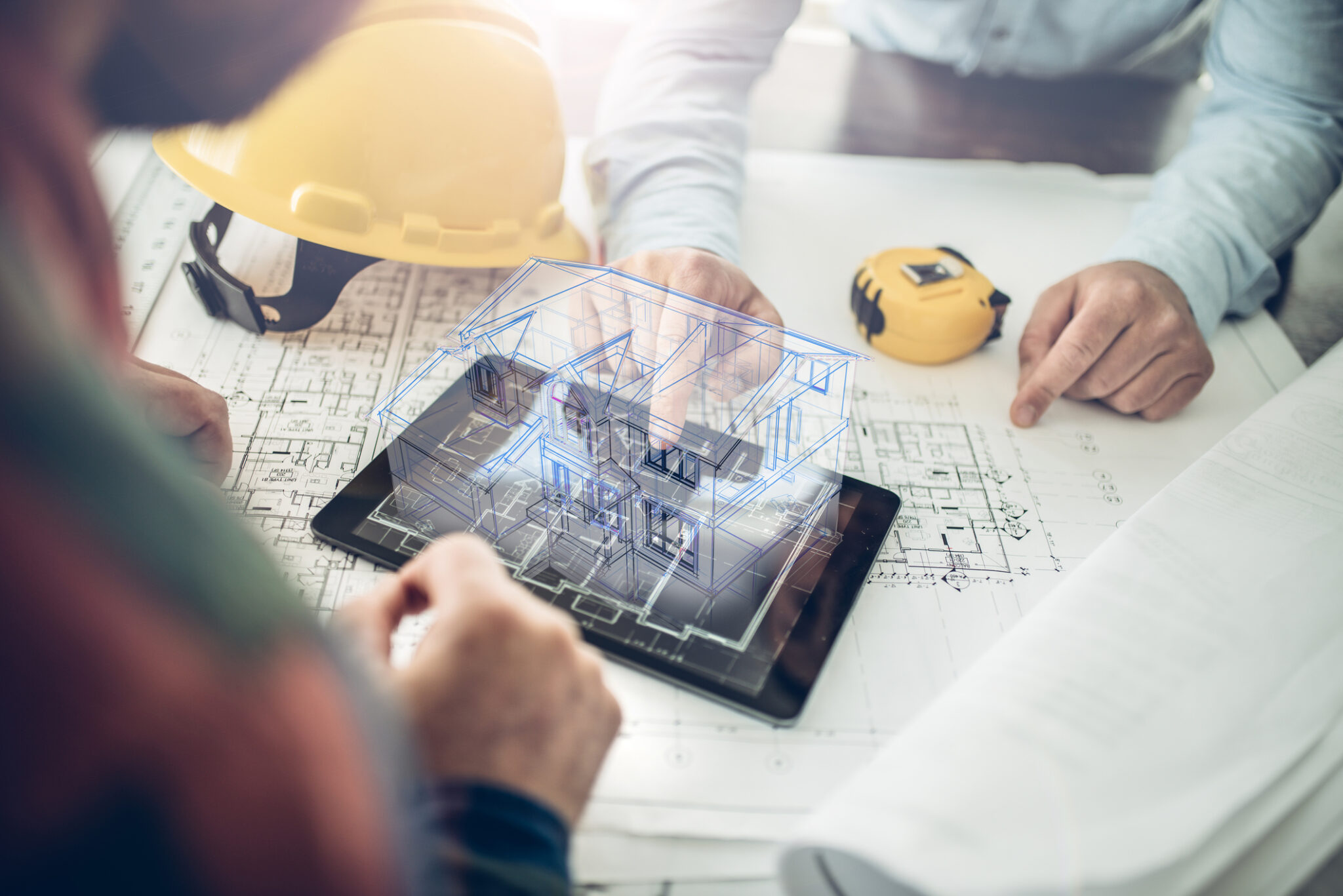
This study hypothesizes that a decision framework can be developed to capture and automate life cycle value evaluation of noise-mitigating measures using multi-criteria decision modeling, building Information modeling and acoustics simulation.
Life Cycle Value Tradeoff of Noise Mitigating Measures using BIM-Simulation and Multi Criteria Decision Modeling: Revisit to Healthy School Environments
Parminder, K. Juneja1
Abstract
Elementary classrooms acoustics plays a critical role in students’ learning, behavioral development, and overall well-being. Studies have consistently shown that the youngest children, less than 13 years age, are the most vulnerable to unfavorable acoustic conditions than adults. Yet environmental noise levels and reverberation time in elementary classrooms during regular school activities are much higher than recommended by WHO or ANSI. Factors that significantly increase the susceptibility of young children to surrounding noise include poorer selective attention ability, underdeveloped reorientation ability, immature linguistic and cognitive development, and temporary hearing impairments, to cite a few key factors. Studies suggest that poor acoustics continue to exist due to lack of acoustics education for architects and engineers; lack of perception of the problem by educators, and lack of sensitivity of the professionals involved during the decision making stage of design.
This study hypothesizes that a robust structured life cycle value evaluation decision framework to facilitate choice of best fit classroom acoustics can be developed and validated. The overarching objective is to capture and automate life cycle value evaluation of noise-mitigating measures using multi criteria decision modeling (MCDM), Building Information modeling (BIM), and acoustics simulation (SIM), such that decisions about classroom acoustics are guided by sound acoustics theories and rationality. The process will make sure that these decisions are not restricted to initial costs only due to cognitive limitations of information processing of human brain. The approach is expected to bridge the gap between ANSI S12.60-2010 standards for classroom acoustics and the real-time practice for acoustical design of classrooms. The work in progress research is discussed and methodology is laid out for the development of life cycle value evaluation model.
Keywords: Acoustics simulation, Building Information Modeling (BIM), Elementary classrooms, Life cycle value assessment (LCVA), Multi criteria decision modeling (MCDM).
Introduction
Elementary classrooms acoustics plays a critical role in students’ learning, behavioral development, and overall well-being (Anderson, 2004; Lubman & Sutherland, 2002). In a multiple noise source environment, human auditory system face significant challenge to distinguish one sound source from another because the acoustical waveforms from all sound sources mix linearly before they reach the ears (Wightman, Kistler, & O’Bryan, 2010). Wolfram (2012) stated that it is easier for adult brains to fill in the meaning of unintelligible, but predictable words from the greater context. However, young children in their early developmental stage lack the ability to discern
from high predictability contexts. The result is impaired language development and acquisition of reading skills (Tamburlini, Ehrenstein, & Bertollini, 2002). The guiding theories behind these results are Colle and Welsh’s (1976) irrelevant speech effect, Jones’s (1993) irrelevant sound effect, LeCompte’s (1995) changing-state hypothesis, Graham’s (1979) orienting reflex, Milgram‘s (1970) cognitive overload model. Noisy surroundings also pose a serious threat to children’s physical and psychological health, and contribute to a reduction in cooperative behavior and trigger aggressive social behavior (Basner et al., 2014; Evans & Lepore, 1993a; Klatte, Hellbrück, Seidel, & Leistner, 2010; Lubman & Sutherland, 2002). The most common negative effects observed are, increased stress and annoyance, reduced motivation, early fatigue, and high blood pressure, to name a few.
In view of the discussed negative impacts of surrounding noise on kids, the American National Standards Institute (ANSI) published ANSI S12.60 (Acoustical Performance Criteria, Design Requirements, and Guidelines for Schools) in 2002, revised in 2010 (American National Standards Institute, 2002). Similarly, World Health Organization (WHO), Australia, France, Japan, Sweden, Italy, and United Kingdom each have either completed or proposed national standard for classroom acoustics (Acoustical Society of America, 2000). These standards are based on a number of studies all around the globe.
Yet after more than a decade, studies around the globe show that the average background noise (BN) levels during regular school activities in classrooms including open classrooms, cellular classrooms, and acoustically treated classrooms vary between 4-38 dB above the WHO guidelines or ANSI recommendations (Ibrahim & Richard, 2000; Kvernstoen, 2007; Picard & Bradley, 2001; Ronsse & Wang, 2013; Shield & Dockrell, 2003; Tamburlini et al., 2002). The longer reverberation time, i.e. greater than 0.6 sec, in these classrooms further adds to the problem by significantly reducing speech intelligibility. Crandell & Smaldino (2000) documented five studies that reported reverberation times in classrooms between 0.4 – 1.2 seconds. Lin, Chaing, Wu, Su, & Guo (2006) reported average school noise measured in 12 schools of sixth-grade children to be greater than 60 dB(A).
It is obvious that despite the wide research, national and international standards for acoustics in classrooms, the elementary school environments are coming short when it comes to acoustical performance of classrooms. Literature suggests many reasons for this shortfall including the problem of what gets measured gets integrated during the cost-cutting business when a new school comes in over budget; and the problem of lack of perception of the issues with the poor acoustics (Thibault, 2005). The objective of this study is to bridge the gap between published acoustic standards for classrooms and the real practice of classroom design and construction. The proposed life cycle value assessment model for classroom acoustics (LCVCA), and its integration with Building Information Modeling (BIM), and acoustics simulation (SIM) is expected to guide decision-makers implementing new standards and policies. It is also expected to provide professionals who are involved with school design and construction with a tool that details decision rules for making informed choices about classroom acoustics with in a specific decision context. The ultimate goal is to develop and validate this robust and structured life cycle value evaluation framework (LCVCA-BIM-SIM) which can be used to facilitate the choice of optimal classroom acoustics during the planning, design, and decision making phase.
In the next section on theoretical background, non-auditory effects of noise on children are discussed in detail followed by a discussion on how MCDM is a valid and flexible approach to address the issue of life cycle value assessment of classroom acoustics at its roots. Methodology to develop and validate LCVCA, and integrate LCVCA with BIM and SIM is discussed followed by future research pathways and conclusions.
Theoretical Background
Children in noisy classrooms
Abundant studies show the detrimental non-auditory impacts of poor classroom acoustics, i.e. noisy soundscapes, on children’s perceptions, academic performance, psychosocial development (social behavior), and overall well-being. The severity of the impact is inversely proportional to children’s age. Gumenyuk, Korzyukov, Alho, Escera, & Naatanen (2004) showed that young children are more impacted by distracting sounds from the environment because ability to resist switching of attention to distracting events and to keep attention focused on the main task or reorient the attention back to the main task is a age related developmental process. In line with the developmental literature (Pearson & Lane, 1990) Gumenyuk et al. (2004), showed a significant correlation between age and auditory reorientation ability, which is not fully developed until 13 years in children.
Picard & Bradley (1997) suggested that background noise (BN) reduces speech intelligibility and increases attentional demands on students. They further explain that this may exert the greatest information processing demands on the youngest children because youngest creates the most BN. Crandell (1993) and Nabelek & Nabelek (1994) discussed that BN reduces speech intelligibility thereby compromising psychoeducational and psychosocial development of kids. Koszarny (1978) suggested that BN tend to affect concentration and attention more seriously in children with lower IQs, which is even more serious effect to consider because lower IQ kids are at a higher risk of getting cognitively tired sooner than higher IQ kids. Lehman & Gratiot (1983) stated that reductions in classroom noise via acoustical modifications significantly improved concentration, attention, and participatory behavior in children.
In the presence of similar BN levels, speech recognition and memory is shown to have a direct correlation with reverberation time. As the reverberation time increases speech recognition and memory is significantly reduced (Kjellberg, 2004; Ljung & Kjellberg, 2009; Ljung, Sörqvist, Kjellberg, & Green, 2009). Reverberation affects speech intelligibility by masking direct and early reflected sound energy by reverberant speech energy. The reverberant speech energy reaches the listener after the direct sound, and overlaps with direct signal which results in smearing or masking of speech (Ljung et al., 2009). BN and reverberation combine synergistically to affect speech perception, i.e., interaction of noise and reverberation negatively affects speech perception to a greater extent than BN or reverberation alone. Crandell, Smaldino, & Flexer (1995) discussed that presence of BN or reverberation alone might reduce listener’s perception of speech by 10%. However, if both BN and reverberation are present in a room, their combine effect might equate to 40 to 50% reduction in speech perception. Reflections fill in the temporal gaps in the noise thereby making it a more steady state in nature thereby creating a completely different sentence or a gibber, like confusing bear for hair. With such errors younger children can easily lose the content of the message due to their inability to take advantage of language context effects (Jerger, Martin, Pearson, & Dinh, 1995).
Other well documented non-auditory impacts of BN on children include: fatigue (Persinger, Tiller, & Koren, 1999), annoyance (Zannin, Zwirtes, & Passero, 2012), botheration (Klatte, Lachmann, & Meis, 2010) and raised blood pressure (Evans & Lepore, 1993b). Klatte et al. (2010) discussed that in noisy environment children are tired sooner, may feel they are treated unfairly by their teacher, are less motivated, overall creating an environment of tension and reluctance to learn. In another study, students aging 13-14 years identified classroom chatter and sound in the hallway as the most annoying source of noise that interfered with their math performance (Boman & Enmarker, 2004). These studies show that there are many causal paths between different sources of background noise, reverberation time, and their varying impacts.
Status quo of elementary classrooms around the globe
Despite a plethora of studies in many fields and from different perspectives, BN levels and reverberation time in elementary classrooms around the globe are far above the standards recommended by ANSI and WHO (American National Standards Institute, 2002). Perego, Bertoni et al. (1996) showed that “the daily sound levels in kindergartens is equivalent to the sound levels generated by a roaring traffic boom and exceeds the maximal value of noise admitted by the WHO to enter the School from the outside, which is 40 Leq dB(A)”. Children in these schools, i.e. higher background noise and larger reverberation time, show higher degree of annoyance as compared to children from quieter schools (Dockrell & Shield, 2004). In a broad study of 78,000 schools, United States General Accounting Office (1995) showed that 21,900 schools or 28.1%, had unsatisfactory or very unsatisfactory environmental noise conditions affecting over 11 million students.
Why noisy classrooms?
It is obvious that despite the wide research, national and international standards for acoustics in classrooms, the elementary school environments are coming up short when it comes to acoustical performance of classrooms. Research suggests that noisy classrooms continue to exist for many reasons including, inadequate acoustics education for architects and engineers, deficient perception of the problem by educators, and lack of sensitivity of the professionals involved with school decision making (Thibault, 2005; Zannin et al., 2012). Architects and engineers could be aware of the importance of good acoustical design in classrooms and other learning spaces, however, these features are often discarded during value engineering or simply removed from the project entirely to meet a predetermined budget based on previous school designs (Thibault 2005). “In 1998, an incredible $7.9 billion was spent on school buildings nationwide. For only a fraction more, all these spaces could have been designed or renovated to provide good listening conditions” (Acoustical Society of America, 2000). The result is perpetuation of substandard acoustical performance where students and teachers end up in classrooms that are either too noisy or highly reverberant. So speech intelligibility becomes a cause of significant concern in these classrooms (Klatte, Lachmann, et al., 2010; Lilly & Wowk, 2010).
As an important next step, studies are needed with a focus on performing lifecycle value, ecological and economic tradeoff, assessment of noise mitigating measures rather than the traditional cost only assessment. The problem involves multiple conflicting criteria, multiple decision makers, multiple stakeholders, and multiple alternatives; therefore, it is a valid problem for applying the theories and principles of multi criteria decision making (MCDM). MCDM offers a structured process wherein different opinions, priorities, and values are considered and tradeoff
evaluated such that results reflect the appropriate priority of each factor considered. The expected MCDM model for classroom acoustics will ensure that the direct (economic) and the indirect (environmental, social, psychological, physiological) costs of noisy classrooms are systematically assessed, by considering interrelations and interdependencies among them, such that an informed tradeoff is made between the ecological impacts and economics of classroom design. The model will guide decision-makers implementing new standards and policies; as well as provide professionals who are involved with school design and construction with a tool that details decision rules for making informed choices about classroom acoustics within a specific decision context. In informed decision making practice, an informed tradeoff analysis is performed for classroom acoustics and the decisions are not restricted due to cognitive limitations of information processing of human brains. The proposed approach is expected to help offset the current practice of initial-cost decision making, because, “the additional cost per new classroom for good acoustical performance is not high, ranging from $1500 to $4500” (Perego et al., 1996). But the problem is that what gets measured gets taken care of during the cost-cutting business when a new school comes in over budget.
Overall, the study is designed to accomplish two key objectives. One, develop and validate a robust multi criteria decision making model for life cycle value assessment of classroom acoustics (LCVCA). This model is expected to facilitate the choice of optimal noise mitigating measures during the planning and design phase. Two, develop a framework to integrate LCVCA with building information model for schools (BIM) and acoustics simulation tool (SIM). This integration will allow quick and easy generation of incessant classroom alternatives using BIM, acoustical property analysis using simulation, and life cycle value assessment using LCVCA. The integrated framework will not only suggest optimized classroom acoustics alternative in a given decision context, but also will inform decision makers of the rationale behind the suggestion in terms of tradeoff made between ecological impacts of noisy classrooms on children and economics of acoustic treatment. Literature reveals that not being able to understand and measure the impacts of noisy and reverberant surroundings on children is the key reason that acoustic treatments are simply removed from the project to meet a predetermined budget based on previous school designs (Thibault 2005)
Methodology
The study is designed to develop and validate life cycle value assessment (LCVCA) model followed by integration of LCVCA with BIM and SIM. An overview of complete work plan is explained in detail in the following sub-sections.
Life cycle value assessment (LCVCA) model development
MCDM is a comprehensive, multidisciplinary systems-analysis methodology to ensure that the direct (economic) and the indirect (ecological) costs of a product or process, noisy classrooms in this study, are systematically and rationally assessed, and the value of the end product is maximized. Typically, financial impacts are included in the systemic evaluation process to reflect both ecological and economic assessments. Depending on the usage, limitations, and uncertainty of a decision context, literature suggests over 70 MCDM techniques including, fuzzy logic, multi attribute utility analysis (MAUA), goal programming, analytical hierarchy process (AHP), ELECTRE, and PROMETHEE, to name a few MCDM methods. All MCDM approaches make the alternatives and their contribution to the different criteria explicit, and all require exercise of
judgment. However, they differ in how they combine the data. Generally, alternatives are first evaluated explicitly with respect to each criterion to obtain criterion specific priority scores. These scores are then aggregated into overall preference values. Despite different MCDM methods, generally MCDM process is similar for all approaches (Dooley, Smeaton, & Ledgard, 2005). A typical MCDM process follows a systematic set of steps (see Figure 1). The methods/techniques that will be used to accomplish each step in this study are described below.
Figure 1. Typical MCDM Process
LCVCA model step 1-Identify criteria and structure hierarchical model/ value tree
The first step in building an MCDM model is always to represent and structure objectives (criterions that are of key importance) that decision makers want to achieve. Montibeller & Franco (2010) suggests “several tools for the structuring of objectives such as, means-ends networks, causal/cognitive maps, post-it workshops with the CAUSE framework, Parnell’s affinity diagrams, and Soft-Systems Methodology”. Cognitive maps will be used in this study to facilitate structuring of objectives and value tree. The representation will be a network representing ends decision makers want to achieve and means available to them, connected by links showing perceived influence (Belton, Ackermann, & Shepherd, 1997; Watson & Buede, 1987). A preliminary work in progress value tree for the problem of classroom acoustics is shown in Figure 2.
Figure 2. Preliminary Value Tree for Classroom Acoustics
This value tree is based on the literature analysis only. As a next step, 30 – 40 experts from various disciplines working in the educational setting, such as reading specialists, regular and special education teachers, teachers of the deaf and hearing impaired, audiologists, speech-language pathologists, and psychologists, will be invited to participate in a cognitive mapping process. The
overall objective would be to design and validate a value tree that addresses the auditory and non-auditory effects of noise on the youngest (k-5) children such that that the problem of better classroom acoustics is addressed using the MCDM approach of decision making? The experts will answer questions such as, “why acoustical performance standards, i.e. background noise levels = 35db, RT = 0.4 seconds, and S/N = +15db, are important for elementary school classrooms?” James’s (1984) within group inter-rater agreement index (rwg) will be used to find consensus among the experts for the criterions and the overall structure of the value tree, where rwg between 0.00 and 0.30 suggests a complete lack of agreement; rwg between 0.31 and 0.50 suggests a weak agreement; rwg between 0.51 and 0.70 implies moderate consensus; rwg between 0.71 and 0.90 implies strong agreement; and, finally, rwg between 0.91 and 1.00 implies very strong agreement.
LCVCA model step 2-Identifying and defining attributes
The structure of a value tree is such that performance of an alternative on the overall objective is measured by its performance on the lowest level criterions. Therefore, all the lowest level, i.e., have a quantitative or qualitative attribute. An attribute can be natural or constructed, and direct or indirect, and should possess the following properties: be comprehensive, unambiguous, operational, and understandable. Many methods exist for defining attributes, such as Parnell’s preference ranking for selecting attributes, Keeney and Gregory’s decision model for selecting attributes, and Kirkwood’s classification of attributes and guidelines for their development (Keeney & Gregory, 2005; Kirkwood, 1997 ; Parnell, 2007). K & G’s (2005) decision model for selecting among the different types of natural, proxy, and constructed attributes will be used for this study because this decision model is well-based in theory and provides comprehensive guidelines for developing attributes. Figure 3 shows the constructed attributes designed to measure noise annoyance and speech recognition in terms of objective acoustical properties of classrooms. Such attributes will be designed and validated for all the lowest level criterions in the value tree for the choice of noise mitigating measures.
LCVCA model step 3 -Selection of MCDM methodology and aggregation rule
Literature suggests more than 70 MCDM methods that have been developed and applied to different decision making problems. Consequently, selection of appropriate MCDM methodology in itself has become a complex decision making problem because use of inappropriate methodology can result in misleading solutions to the decision making problem. One of the most important requirements of a method to be used in selection of noise mitigating measures or acoustics design, is that it should be quick, simple, and easy to use and understand. Many of the MCDM methods involve quite complicated mathematics, thus they do not appear to have the user-friendliness and transparency that is required for use in classrooms acoustics design. In view, Eldrandaly, Ahmed, & AbdelAziz (2009) descriptive framework to select the most appropriate MCDM technique for a given decision context will be used for this study. The framework
recommends a set of MCDM techniques based on the characteristics of the decision problem, the decision maker, and the solution technique.
Typically in MCDM models, a local preference for each criterion is aggregated to find the overall value of each alternative. To this end, validated OWA (ordered weighted averaging) or OWG (ordered weighted geometric) operators for aggregation will be employed depending on the additive or multiplicative characteristics of the criterions (Cheng & Chang, 2006).
LCVCA model step 4 – Identify criteria weights
In most MCDM problems, the criteria weights play an important role in estimating the overall preference values of alternatives. Usually weights represent marginal contributions, criteria trade-offs, scaling factors, and criteria importance (Keeney, 1996). Depending on the MCDM aggregation rules, which use the criteria weights in different ways, many methods for eliciting criteria weights have been suggested in the literature. Choo & Wedley (2008) identified a diverse array of plausible interpretations for the criteria weights such as “marginal contribution, indifference trade-offs or rates of substitution, gradient of the overall value function, scaling factors converting into commensurate overall value, relative contribution of the average criterion specific scores, discriminating power of the criteria on the alternatives, relative contribution of swing from worse to best on each criterion, vote values in binary choices, relative contribution of the criteria at the optimal alternative, relative functional importance of the criteria.” Choo, Schoner, & Wedley (1999) determined the appropriate questions that should be posed to the DM for eliciting information on criteria weights. These questions will be used in this study to elicit weights for each criterion from the experts from step 1. The experts will be well-informed about the exact reason for obtaining the criteria weights. Such as in MAUT, Keeney and Raiffa (1976) underlines that the attribute weights are not importance weights for attributes; rather, they are rescaling factors that provides consistency to the overall multi-attribute utility evaluation i.e., weights express the relative contribution of one attribute to the overall evaluation of alternatives.
The individual criteria weights provided by the experts will be aggregated to obtain group weights for all criteria. According to Saaty (1995) for a group of decision makers taking the geometric mean of weights is the most appropriate method to obtain group weight. The geometric mean of group of weights is calculated as shown in equation 1 (Saaty, 1995):
(1)
Where Wj is the weight of jth criterion, w1,j -----wm,j is the weights provided by m decision makers to the jth criterion.
The identification of criteria weights will culminate the MCDM process for LCVCA model development. The next step in any MCDM process, as shown in Figure 1, typically involves generation of alternatives and alternative performance evaluation in regards to the overall objective of the decision problem. These steps are discussed in detail in the next section.
LCVCA Model Application
LCVCA model step 5 -Noise mitigating alternatives for classroom acoustics
Generation of alternatives is critical to effective design process and MCDM evaluation (Andresen, 2000; Ozernoy, 1985). In other cases time and resources is wasted on evaluating alternative
solutions that are far from fulfilling goals of the stakeholders. Generating alternatives is an art, an exercise of creativity and experience. ANSI standards and research has already established optimal values for sound levels, RT and S/N ratio in classrooms. These standards will be chosen as the best alternative and four alternatives will be generated with varying distance from the best alternative. Design trade-offs between the various criteria will be chosen based on the building systems driving the basic values of the criteria. Background noise level is determined by two main building systems, the envelope and the HVAC. School siting is important in deciding a strategy, since external conditions drive internal noise levels. For example, increasing budget for a low noise HVAC installation may produce no change in environment at a location with high external noise levels and a low budget envelope. Lowering reverberation times without decreasing background noise would also not produce an effect on the final acoustical state of the room. Much of this work will depend on the classroom architectural standard employed by regional school construction authorities.
LCVCA model step 6&7-Performance evaluation of alternatives
Performance of each alternative on each criterion will be measured using the attribute scale identified in Step 2. The aggregation model identified in step 3 will be used to evaluate the overall performance of alternative solutions. The variant which is awarded the highest overall performance should be the best one in a given decision context.
LCVCA Model Validation
The concept of criterion related validity also referred to as instrumental validity or predictive validity will be employed to test the validity of the results of the LCVCA model. Criterion validity shows the accuracy of an instrument or a device by comparing it with another measure or a procedure that has been shown to be valid (Heffner, 2004), or another measurement obtained from the same target population (Carmines & Zeller, 1979). We will compare the rankings of the alternative classroom acoustics (obtained in step 7) with the known values of these alternatives identified in step 5 as known distance from the optimal.
Criterion validity is the degree to which the measurement correlates with an external known criterion or another measurement obtained from the same target population. A correlation coefficient of zero implies there is no relationship between the measured or predicted values and the direct measurement. As the strength of the relationship between the measured or predicted values and direct measurement increases, so does the correlation coefficient. A correlation coefficient of 1 implies that there is a perfect correlation between the measured value and a known value.
An illustration of how the LCVCA would be used by the decision makers during the planning or decision making stage is shown and discussed in the next section on practical application.
Practical Application-LCVCA Illustration
An illustration for LCVCA is shown in Figure 4. Two alternatives are considered A1 and A2, where A1 represents the best possible scenario in terms of short reverberation time (RT – < 0.4 seconds) and minimal background noise (35–40dB); and A2 is at a significant distance from A1 on the negative side with higher RT and higher background noise. For ease of presentation, equal weighing is used in this illustration. The scores of alternatives A1 and A2 on each criterion are
shown in oval shapes. The simplest method of aggregation for overall value, i.e. additive weighing, is shown to illustrate the application of the LCVCA to choice of noise mitigating measures. As per MCDM, the alternative with the highest overall value, A1 in this scenario, is the best option to adopt in a respective decision context.
BIM-Acoustics Simulation-LCV-CA Integration
The follow up will involve building a decision support system prototype application, called the Acoustics advisor for classrooms (AACR). AACR will shift the development focus from the domain expertise to the client or users of that expertise; that is, from the knowledge base to the input/output interface. The acoustics advisor will provide recommendations using the value tree (step1), the attributes (step 2), the weights (step 3), and the MCDM aggregation (step 4) that are the knowledge base of the decision model. The output will be ranking or rating of classroom alternatives with different noise mitigating measures. The Acquire ActiveX control gives a quick and easy way to create intelligent applications using Microsoft's Visual Basic and other software development systems. This decision support system could be adopted by all school decision boards to make informed decisions about classroom acoustics for new schools or retrofitting existing schools to accomplish the key objective of any learning environment – cognitive growth and, psychological, psychosocial well-being.
To fine tune the process further, AACR is expected to communicate with Building Information Modeling (BIM) and acoustics simulation tool (SIM). Figure 5 shows the overall procedure for this integration. IFC file from school’s BIM model will be imported into simulation tool that directly calculates acoustical values of classroom such as, reverberation time (RT) and early decay time (EDT). It also calculates expected sound pressure levels (SPL) based on the source having a sound power representative of speech. These acoustical properties are then sorted and fed into AACR to calculate the overall performance of each alternative. The output is shown as life cycle value and ranking of alternative scenarios (Figure 5).
Integration of school’s BIM model with AACR provides an opportunity to create and analyze many what-if scenarios during policy development, planning, and design stage. This integration is also expected to improve the way data is utilized in a building throughout its life cycle, and to model impact of design and decisions made in an effort to improve classrooms’ contribution to the
objectives of learning, psychological, physiological, and positive social development. Pertinent information contained within a BIM model for a classroom will be extracted, and utilized in decision-making related to selection of noise mitigating measures, their operations and maintenance, and the development of ‘what-if’ scenarios, such that the decision makers can literally see the implications of ignoring acoustical performance of classrooms. The research would result in a new means for sharing data amongst various building modeling programs, and a new tool for making policy and design decisions related to acoustics design for elementary classrooms.
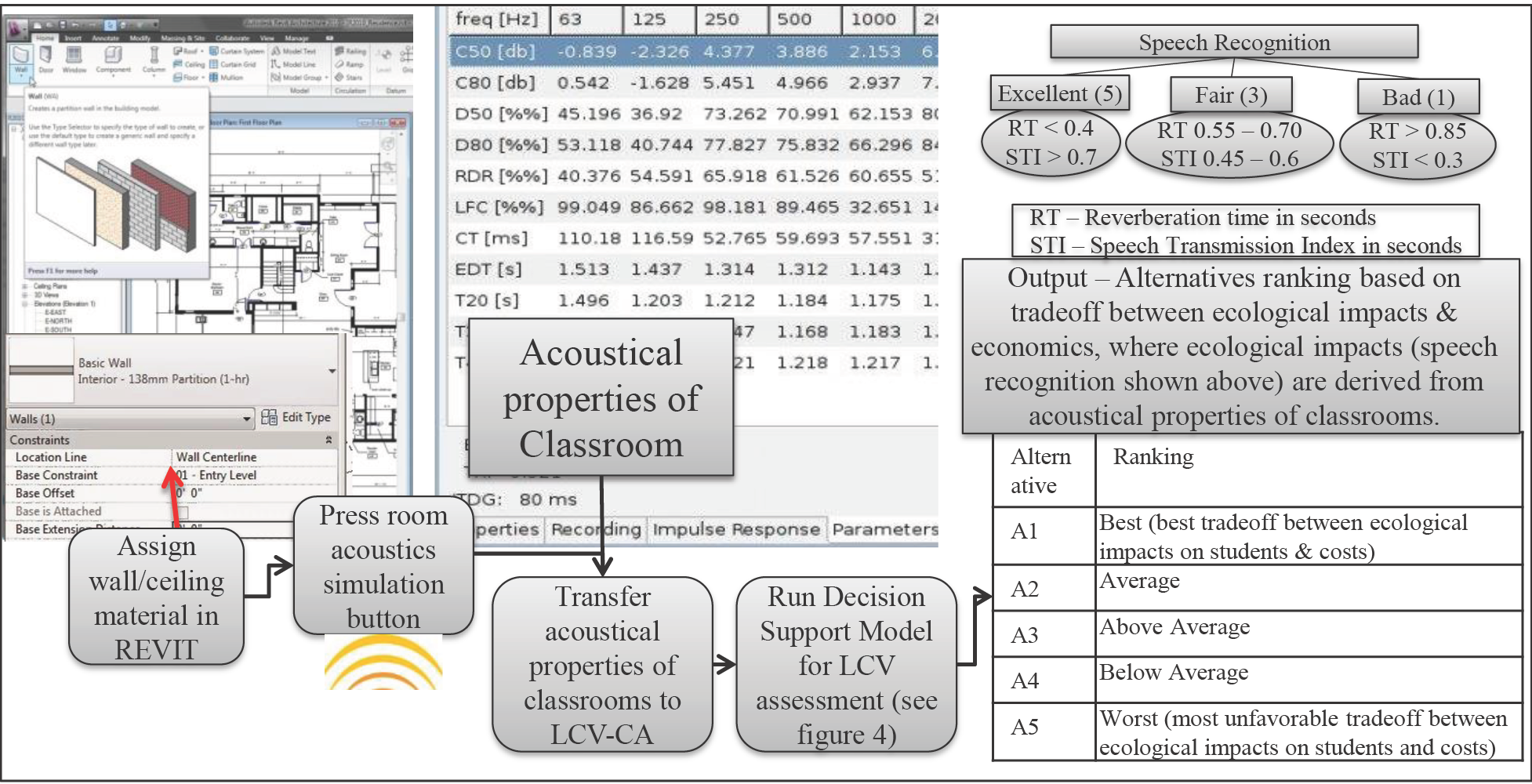
Figure 5. Proposed Integration framework for LCVCA-BIM-SIM
Future Research
The comprehensive approach developed in this study could be extended to different indoor environmental variables that are shown to have a significant correlation with occupant’s productivity and overall well-being. These indoor environmental variables include, temperature, humidity, air quality, natural light, and natural view, to name a few. The overarching objective would be to facilitate decision makers and policy creators in making informed decision about school’s indoor environment such that life cycle value of classrooms is maximized by maximizing students’ learning, behavioral development, and overall well-being. The approach is in strategic alignment with triple bottom line for schools – economy, environment, social.
Conclusions
Noise for kids is a well-researched scientific problem. Despite the negative bearings of noise on kids, research shows that elementary schools all over the globe show noise levels much higher than prescribed by the acoustical standards. Youngsters who are the most vulnerable to noise in their surroundings are growing and learning in environments that are far below the standards suggested by researchers and institutions all over the world.
Research also shows that implementation of acoustical standards to address the problem of noise in elementary schools is the key problem area that needs attention. Typically, school board of directors does not include an acoustical consultant or an audiologist. These adults who are the key decision makers are either unaware of the negative impacts of noise on kids or they are unable to perceive how noise negatively impacts young children. Therefore, when a new school comes in over budget the acoustical treatments goes out of the window because what gets measured gets integrated during the cost-cutting business. Cost of acoustical treatments is easily measurable whereas measurement of negative impacts of noise on younger children will require more time, money, and expertise.
The proposed conceptual framework is a novel approach to address the issue of poor acoustics in elementary school classrooms. The LCVCA-BIM-SIM framework for classroom acoustics is expected to guide decision-makers implementing new standards and policies as well as provide professionals who are involved with school design and construction with a tool that details decision rules for making informed choices about classroom acoustics with in a specific decision context. The model will facilitate effortless generation of various possible alternatives and what-if scenarios so that decision makers can virtually see the implications of ignoring establishment of optimal acoustical conditions. In addition, this integration will meet the future quality assurance needs of the building industry where each building from its conception to its useful life will be captured as a BIM model.
Furthermore, the LCVCA model is expected to provide benefits throughout the life cycle of elementary school classrooms from policy making to designing and operating. By providing a comprehensive picture, the MCDM approach ensures that a school doesn't create improvement in one area at the expense of another. Rather than looking at specific indicators in isolation, MCDM analysis is the most appropriate approach to quantify and compare the ecological and economic tradeoff together in one model over the life of a product. Moreover, the better acoustical performance building envelopes that result from the process produce energy savings over the life of the building.
References
Acoustical Society of America. (2000). Classroom Acoustics I – A resource for creating learning environments with desirable listening conditions. Melville, NY: Acoustical Society of America.
American National Standards Institute. (2002). Acoustical Performance Criteria, Design Requirements, and Guidelines for Schools ANSI S12.60-2002 (pp. 1 -36). Melville, New York: Acoustical Society of America.
Anderson, K. (2004). The Problem of Classroom Acoustics; The Typical Classroom Soundscape is a Barrier to Learning. Seminars in Hearing, 25(2), 117 -129.
Andresen, I. (2000). A Multi-Criteria Decision-Making Method for Solar Building Design. Norwegian University of Science and Technology.
Basner, M., Babisch, W., Davis, A., Brink, M., Clark, C., Janssen, S., & Stansfeld, S. (2014). Auditory and non-auditory effects of noise on health. (383).
Belton, V., Ackermann, F., & Shepherd, I. (1997). Integrated support from problem structuring through to alternative evaluation using COPE and VISA. Journal of Multi-Criteria Decision Analysis, 6, 115-130.
Boman, E., & Enmarker, l. (2004). Factors affecting pupils’ noise annoyance in schools: The building and testing of models. Environment and Behavior, 36, 207-228.
Carmines, E. G., & Zeller, R. A. (1979). Reliability and validity assessment. Beverly Hills London: Sage Publications.
Cheng, C.-H., & Chang, J.-R. (2006). Mcdm Aggregation Model Using Situational Me-OWA and Me-Owga Operators. International Journal of Uncertainty Fuzziness and Knowledge-Based Systems, 14(4).
Choo, E., & Wedley, W. C. (2008). Comparing fundamentals of additive and multiplicative aggregation in ratio scale multi-criteria decision making. The Open Operational Research Journal, 2(1), 1-7.
Choo, E. U., Schoner, B., & Wedley, W. C. (1999). Interpretation of criteria weights in multicriteria decision making. Computers & Industrial Engineering 37 (1999) 527±541, 37, 527-541.
Colle, H. A., & Welsh, A. (1976). Acoustic Masking in Primary Memory. Journal of verbal learning and verbal behavior, 15(1), 17-31.
Crandell, C., Smaldino, J., & Flexer, C. (1995). Sound field FM amplification: Theory and practical applications. San Diego, CA Singular Press.
Crandell, C. C. (1993). Speech recognition in noise by children with minimal degrees of sensorineural hearing loss. Ear Hear, 14(3), 210-216.
Crandell, C. C., & Smaldino, J. J. (2000). Classroom Acoustics for Children With Normal Hearing and With Hearing Impairment. Language, Speech, and Hearing Services in Schools, 31, 362-370.
Dockrell, J. E., & Shield, B. (2004). Children's Perceptions of their Acoustic Environment at School and at Home. Journal of the Acoustical Society of America, 115(6), 2964 -2973. doi: 10.1121/1.1652610
Dooley, A. E., Smeaton, D., & Ledgard, S. F. (2005). Identification Of Important Criteria In Farm. Systems Decisions Around Lake Taupo. Paper presented at the New Zealand Agricultural and Resource Economics Society (NZARES), Tahuna Conference Centre – Nelson, New Zealand.
Eldrandaly, K., Ahmed, A. H., & AbdelAziz, N. (2009). An expert system for choosing the suitable MCDM method for solving a spatial decision problem, in 9th International Conference on Production Engineering, Design and Control, PEDAC. 2009: Alex, Egypt. Paper presented at the 9th International Conference on Production Engineering, Design and Control, PEDAC Alexandria, Egypt.
Evans, G. W., & Lepore, S. J. (1993a). Non-auditory Effects of Noise on Children: A Critical Review. Children's Environment, 10(1), 42 -72.
Evans, G. W., & Lepore, S. J. (1993b). Nonauditory effects of noise on children: A critical review. Children's Environments, 10(1), 31-51.
Graham, F. K. (1979). Distinguishing among orienting, defense, and startle reflexes. In H. D. Kimmel, E. H. V. Olst & J. F. Orlebeke (Eds.), The Orienting Reflex in Humans (pp. 137-167). New Jersey Hillsdale.
Gumenyuk, V., Korzyukov, O., Alho, K., Escera, C., & Näätänen, R. (2004). Effects of auditory distraction on electrophysiological brain activity and performance in children aged 8–13 years. Psychophysiology, 41, 30-36.
Heffner, C. L. (2004). Research Methods Retrieved from http://allpsych.com/researchmethods/
Ibrahim, Z. H., & Richard, H. K. (2000). Noise Pollution at School Environment Located in Residential Area. Journal of Civil Engineering, 12(2), 47-62.
James, L. R. (1984). Estimating within-group interrater reliability with and without response bias. Journal of applied psychology, 69, 85-98.
Jerger, S., Martin, R., Pearson, D., & Dinh, T. (1995). Childhood hearing impairment: auditory and linguistic interactions during multidimensional speech processing. Journal of speech and hearing research, 38(4), 930–948.
Jones, D. M. (1993). Objects, streams and threads of auditory attention. In A. D. Baddeley & L. Weiskrantz (Eds.), Attention: Selection, awareness and control. Oxford: Clarendon Press.
Keeney, R. L. (1996). Value-Focused Thinking: A Path to Creative Decisionmaking: Harvard University Press.
Keeney, R. L., & Gregory, R. S. (2005). Selecting Attributes to Measure the Achievement of Objectives. Operations Research, 53(1), 1-11.
Keeney, R. L., & Raiffa, H. (1976). Decisions with Multiple Objectives: Preferences and Value Trade-Offs New York: Wiley.
Kirkwood, C. W. (1997 ). Strategic Decision Making: Multiobjective Decision Analysis with Spreadsheets. Belmont, CA: Duxbury Press.
Kjellberg, A. (2004). Effects of reverberation time on the cognitive load in speech communication: theoretical considerations. Noise & Health, 7, 11-21.
Klatte, M., Hellbrück, J., Seidel, J., & Leistner, P. (2010). Effects of Classroom Acoustics on Performance and Well-Being in Elementary School Children: A Field Study. Environment and Behavior, 42(5), 659 -692. doi: 10.1177/0013916509336813
Klatte, M., Lachmann, T., & Meis, M. (2010). Effects of noise and reverberation on speech perception and listening comprehension of children and adults in a classroom-like setting. Noise Health, 12(49), 270-282.
Koszarny, Z. (1978). Effects of aircraft noise on the mental functions of school children. Archives of acoustics, 3, 85-86.
Kvernstoen, R. A., INC. (2007). Classroom Acoustical Study.
LeCompte, D. C. (1995). An irrelevant speech effect with repeated and continuous background speech. Psychonomic Bulletin & Review, 2(3), 391-397.
Lehman, A., & Gratiot, A. (1983). Effects du bruit sur les enfants a l’ecole. Paper presented at the 4th Congress on Noise as a Public Health Problem Milano: Centro Ricerchee Studi Amplifon.
Lilly, J. G., & Wowk, R. (2010). Acoustical performance standards for schools.
Lin, Y. C., Chaing, C. M., Wu, J. L., Su, H. J., & Guo, Y. L. (2006). Noise exposure in taiwanese school children and disturbance on speech comprehension. Epidemiology, 17(6), 171-172.
Ljung, R., & Kjellberg, A. (2009). Long Reverberation Time Decreases Recall of Spoken Information. Building Acoustics, 16(4).
Ljung, R., Sörqvist, P., Kjellberg, A., & Green, A.-M. (2009). Poor Listening Conditions Impair Memory for Intelligible Lectures: Implications for Acoustic Classroom Standards. Building Acoustics, 16(3), 257-265.
Lubman, D., & Sutherland, L. C. (2002). The role of soundscape in children’s learning. Journal of Acoustical Society of America, 112.
Milgram, S. (1970). The Experience of Living in Cities. Sciences, 167(3924), 1461-1468.
Montibeller, G., & Franco, A. (2010). Multi-criteria decision analysis for strategic decision making. 103, 25-48. doi: 10.1007/978-3-540-92828-7_2
Nabelek, A., & Nabelek, I. (1994). Room acoustics and speech perception. In J. Katz (Ed.), Handbook of Clinical Audiology (pp. 624–637).
Ozernoy, V. M. (1985). Generating Alternatives in Multiple Criteria Decision Making Problems: A Survey. Decision Making with Multiple Objectives lecture Notes in Economics and Mathematical Systems, 242, 322-330.
Parnell, G. S. (2007). Value-focused thinking in methods for conducting military operational analysis. In L. Rainey & A. Loerch (Eds.), (pp. 619-656). Alexandria, VA: Military Operations Research Society.
Pearson, D. A., & Lane, D. M. (1990). Reorientation in Hyperactive and Non-Hyperactive Children: Evidence for Developmentally Immature Attention? In A. S. Benjamin, J. S. D. Belle, B. Etnyre & T. A. Polk (Eds.), Advances in Psychology (Vol. 69, pp. 345-363).
Perego, L., Bertoni, G., Goglio, F., & 3, G. G. (1996). Children and noise European Journal of Epidemiology, 12, 549-550
Persinger, M. A., Tiller, S. G., & Koren, S. A. (1999). Background sound pressure fluctuations (5 DB) from overhead ventilation systems increase subjective fatigue of university students during three-hour lectures. Perceptual and Motor Skills, 88, 5.
Picard, M., & Bradley, J. (2001). Revisiting speech interference in classrooms. Audiology, 40(5), 221-244.
Picard, M., & Bradley, J. S. (1997). Revisiting speech interference by noise in classrooms and remedial solutions. Theory & Practical Applications. San Diego, CA: Singular.
Ronsse, L. M., & Wang, L. M. (2013). Relationships between unoccupied classroom acoustical conditions and elementary student achievement measured in eastern Nebraska. Journal of Acoustical Society of America, 133(3), 1480-1495.
Saaty, T. L. (1995). The Analytic hierarchy process for decision in a complex world. Pittsburg: RWS Publicatio.
Shield, B. M., & Dockrell, J. E. (2003). The Effects of Noise on Children at School: A Review. Journal of Building Acoustics, 10(2), 97 -106.
Tamburlini, G., Ehrenstein, O. S. v., & Bertollini, R. (2002). Children’s health and environment: A review of evidence (Vol. 29): World Health Organization.
Thibault, L. (2005). Classroom Acoustics -Hands & Voices Communication Considerations A-Z: US Access Board.
United States General Accounting Office. (1995). School Facilities: Condition of America’s Schools.
Watson, S. R., & Buede, D. M. (1987). Decision Synthesis. Cambridge: Cambridge University Press.
Wightman, F. L., Kistler, D. J., & O’Bryan, A. (2010). Individual differences and age effects in a dichotic informational masking paradigm. Journal of Acoustical Society of America, 128(1), 270-279.
Wolfram, E. P. (2012). lassroom Acoustics. The classroom serves as the acoustic venue for primary and secondary education.
Zannin, P. H. T., Zwirtes, D. P. Z., & Passero, C. R. M. (2012). Assessment of acoustic quality in classrooms based on measurements, perception, and noise control. In D. Siano (Ed.), Noise Control, Reduction and Cancellation Solutions in Engineering. Online: InTech.
Want even more Content?
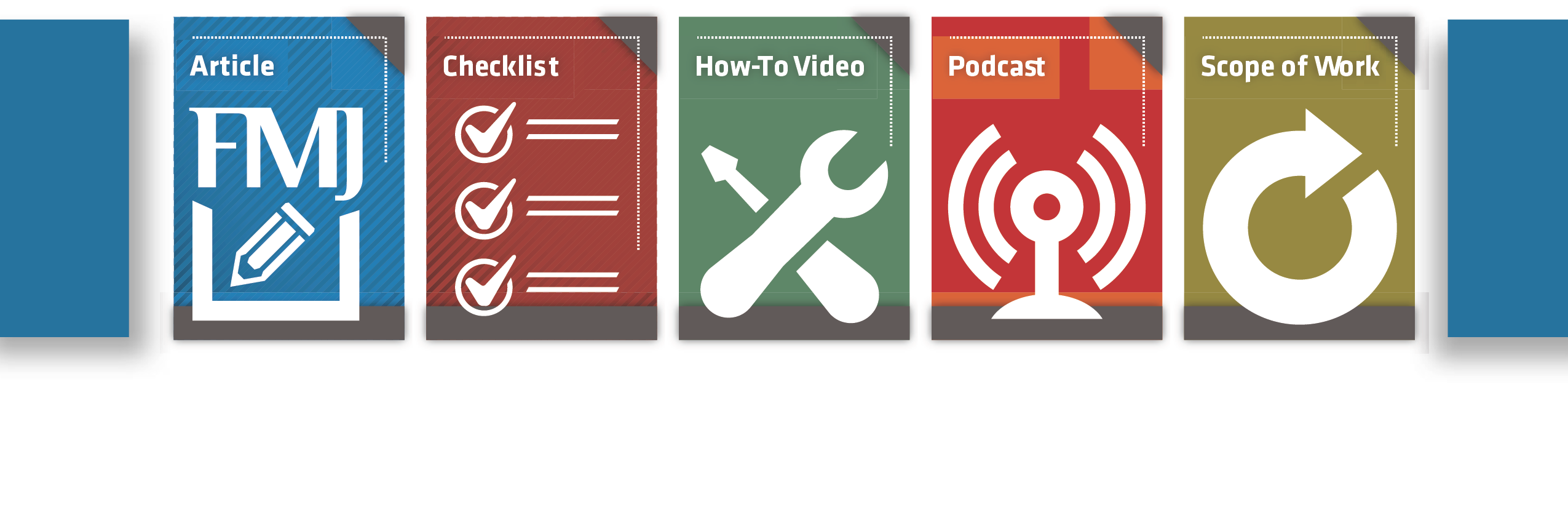
Since you’re already a user, you know that IFMA’s Knowledge Library offers all FM content in one place. But did you also know that by signing up via email to become a registered user, you can unlock even more resources?
Signing up via email for registered access within the Knowledge Library brings more content and functionality to your fingertips. Expect to grow your facility management knowledge, career and network faster than ever before.